RESEARCH
BENEFITS OF LA RESEARCH
LA provides insights into students’ learning processes and gaps in understanding. This can help teachers recognise weaknesses in learning activities and challenging topics, as well as provide constructive feedback that can enhance students’ further learning (Gašević et al., 2015). As for students, LA plays a significant role in fostering self-regulated learning (Schumacher & Ifenthaler, 2018) as an active process in which students set their learning goals and then “monitor, regulate, and control their cognition, motivation, and behaviour” (Pintrich, 2000, p. 453). An area of particular research interest has recently been related to assessment analytics (Nguyen et al., 2017; Divjak & Maretić, 2017) In higher education, analytics often aims at early identification of students at risk, as a basis for interventions aimed at maximizing student retention, and enhancing the quality of students’ learning experiences (Susnjak et al., 2022).
Some of the key goals of LA include providing students with personalised and timely feedback on their learning, and supporting awareness through self-reflection; supporting the quality of learning and teaching with evidence on the success of innovations; supporting students in development of lifelong learning skills and strategies, as well as skills like critical thinking, collaboration, creativity, communication (SOLAR, n. d.).
LA can have different levels of complexity, supporting informed decision-making by providing several layers of insights ( Susnjak et al., 2022; SOLAR, n. d.) :
- descriptive analytics provides basic information about trends and current status
- diagnostic analytics looks into why something happened
- predictive analytics provides forecasts and estimates future outcomes (based on past and current patterns)
- prescriptive analytics produces tailored recommendations and suggestions for behavioural changes which could lead to positive outcomes.
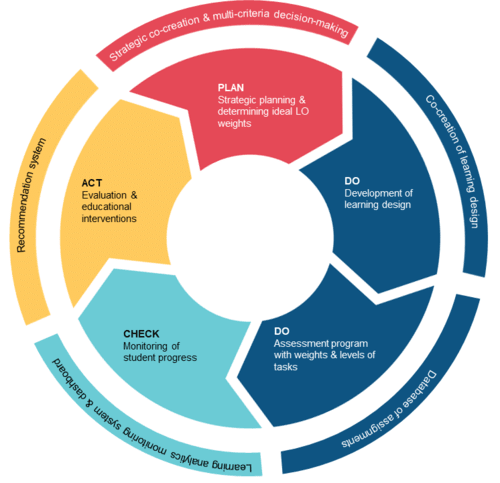
LAB'S RESEARCH PLAN
The LA Lab's team continuously and closely follows the developments related to LA research, which is reflected in our research plan. Our most current research endeavors are primarily related to learning design and design analytics, assessment analytics, developing predictive LA models, and the trustworthiness of LA and AI in education. However, our research plan is much broader, and includes a range of related topics.